
08 January, 2021 0 Comment(s)
Customer Data in Banking
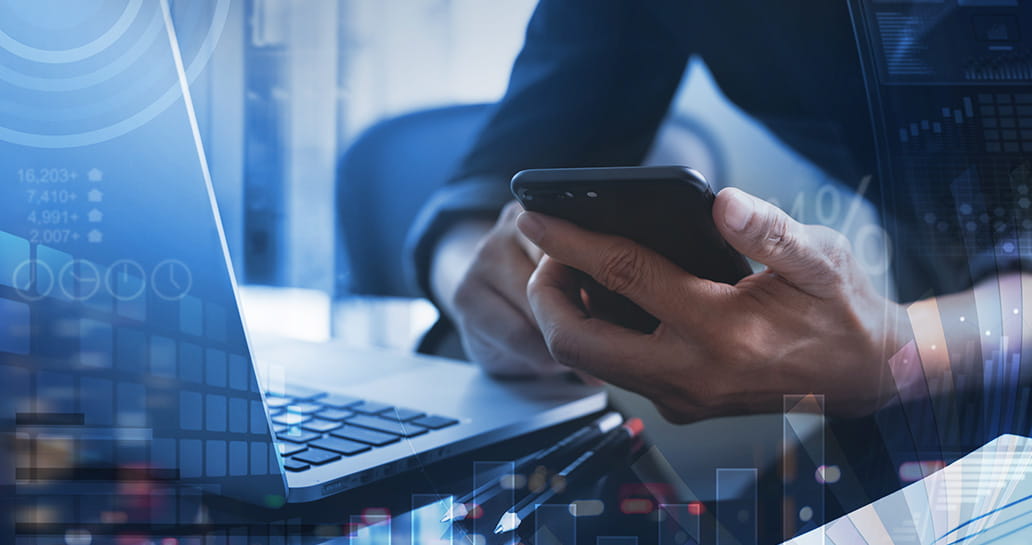
5 Steps to getting your Customer Data sorted
Reference data, sometimes referred to as ‘master data’ or ‘static data’, is fundamental to financial trading, with an estimated 70% of data used in financial transactions being reference data*.
Reference data encompasses a range of details such as a unique security number for the financial instrument or product being traded, the name of the buyer, seller, or any other party involved in the contract, and the costs involved in the trade. Reference data can become far more detailed however, sometimes including information regarding the complex conditions of the trade and facts about all the relevant parties involved.
Reference data is also divisible into two essential types:
- Static data – this type of data won’t change throughout the course of the transaction, and includes specifications such as the names of the parties contracted in the financial transaction (ie. ‘counterparties’) and those in the financial supply chain, as well as information related to the financial products involved, such as their expiration dates or type.
- Dynamic data – information that changes throughout the course of the transaction, such as credit ratings or the pricing of the instrument at different times or at the close of the deal.
Practically speaking, reference data can be understood as boundary conditions required to undertake a transaction or trade in the financial arena, with correct data contributing to an environment for smooth transactional flow. Reference data is continuously being accessed and altered by systems linked to trading desks, risk departments, middle and back offices in financial institutions, with new data usually being added constantly throughout the trade. It is important to protect this static reference data (or Master Data as it is commonly called) and create a single version of truth.
Many of Banking and Financial Customers struggle to ensure that their data is easy to find, current, accurate and shared with only those who need it. A whopping 80% of an analyst’s time is spent solely on discovering and preparing data, according to the Harvard Business Review. At the same time, having unnecessary information on-hand makes it more challenging to locate the information that you do need. Fortunately, these problems can be addressed by implementing Customer Master Data Management (MDM). MDM creates a common definition and a single view of the customer by centralising data and creating governance, compliance and security. Given the amount of data and the number of systems that may be involved, MDM projects can be daunting. So where do you begin? Let’s take a look at the five steps to customer master data management success.
An MDM project’s starting point will depend on where the business falls in the enterprise data management maturity model. Every organisation may be at a different stage and very few are starting from scratch or have only one existing system/application/master list. Here are a few common scenarios:
- Lines of business have been focused on their products and services only, so they aren’t sharing client data with other departments and divisions;
- A company has great data, but has poor governance, so data gets duplicated and you end up with out-of-sync data;
- M&A activity has resulted in an accumulation of data from different firms—but this data has not yet been integrated; and
- A company has grown so quickly that it has outpaced its system’s ability to keep up with its business needs.
Step 1: Identify your customer data and who accesses it
The first order of business is to determine exactly where all of your customer data is located. You will also want to understand which systems actively create or update customer data—versus which systems are passively using data or reporting from it.
Step 2: Create a common definition of a “customer”
“How many customers do you have?” is a pretty basic question, but coming up with an accurate answer can be difficult if you lack a common definition. To get a single definition (and ultimately, a single view) of the customer, you will need to understand how every department of your business views your customers. The retail banking division may count every address as a different customer, but home loans may have multiple addresses or different “customers” may share the same address. A different line of business may define customers as individuals who have made a transaction within the past year. Whatever the case, you must understand your company’s definition of a customer.
The idea is to identify data attributes that will be common to each customer. Establishing a customer’s defining data points is the start of developing a master data model. Well-executed data models define who your customer is in terms that may be used in all lines of business. They also establish the blueprint for how your customer’s data will be organized, so that various attributes may be searched as needed (e.g., you would have the ability to query a list of customers who have made a purchase within the past six months).
Step 3: Ensure data accuracy and eliminate duplicate data
After you have a master data model, you’ll want to ensure that your data is accurate and free of duplicates. If you have customer information in multiple systems, chances are good that you have duplicate customers with mismatching information. You need to correct inaccuracies and create a process to remove duplicate data when you start the integration process.
Step 4: Create a governance program
Governance will help you control your master data model, enabling you to keep your data set clean and accurate. Good governance ensures that your data can be trusted and it provides accountability as you strive to keep it up to date and control access to the data.
Step 5: Implement Master Data Management (MDM)
When your data is clean, and you have a master data model and governance program in place, you can begin with the MDM implementation process.
Additional MDM project considerations
The goal of MDM is to create a single place for all your customer information; in other words, a single data source that you can use to inform your business decisions. If you consider the workings of customer relationship management (CRM) systems, the value of MDM becomes clear. Companies think, “If we can just get the CRM portal up and running, we’ll be able to organize the data over time.” However, without a solid understanding of customer data and a strategy for how it will be managed, consolidating CRMs can compound the problem rather than solving it; it creates yet another system with its own data repository and governance rules.
Bringing It All Together with DDC MDM360
Being in an industry that is getting transformed, are you and your team receiving more business questions that require deep analytics with short turnaround times? Do you grapple with data silos and a lack of tools to bridge those silos and foster collaboration among analytics team members?
DDC MDM360 helps business discover and merge data from multiple disparate databases, making it possible to discover single version of truth that would otherwise go undetected. With robust integrations with a wide range of data sources and Business Intelligence and Data Science tools that your team relies on every day, DDC MDM360 serves as a hub that can make identifying your customer better than ever.